Written by Michael Zargham.
And other musings on algorithmic policy-making
I met the Aragon founders at ETH Buenos Aires May 2018, hung out with the
Chris Burniske,Lane Rettig,Rhys Lindmarkamong many others. I had spent late 2017 and early 2018, wrapping up a paper on the mathematical frameworkI use for designing mechanisms in dynamical systems, and working withMarkus Buhatem KochandFernando Martinelli, applying that framework to complete the proofs underpinning theBalancer Protocol. Notably, the Balancer protocol calls for subjective parameter choices in the form of tokens supported, and weights of pools. It was already clear to many active researchers that governance of parameters was not a hypothetical problem, but one which would eventually come to dominate the political landscape of blockchain-based ecosystems.
Two months later, I attended Tech Crunch Blockchain in Zug July of 2018 and token’s role in governance was a very common conversation point. Feeling motivated, I pulled an all nighter in Zug and wrote my first conviction voting PoC, an algorithm+simulation by framing governance as dynamic average consensus. Note that this was for continuous parameter selection not discrete choices. While in Zug, I met
Griff Green(founder ofGiveth), someone I’ve come to respect greatly — nobody I’ve met in the crypto space has been more giving, a one-man proof that you cannot reduce all humans to selfish agents.

Later that July, I presented my ongoing work at the Ninth Annual Conference on Complex Systems, in Cambridge Massachusetts. There I met Frank Wang, a partner at the research firm X-Order. Over the summer, I continued to mess around with variations of conviction voting; one of the use cases that drove my explorations was the (dynamic) selection of generative art algorithms for Artonomous, a tech-art project by
Simon de la Rouviere. At Frank’s request, I drafted a proposal for continued research on conviction voting which contained the description of governance as“sensor fusion” which included formalizations for both the discrete choice (as in Artonomous) and continuous parameter selection as a collective preference estimation problem.

A few months later in Prague for Devcon IV, Griff introduced me to
Jeff Emmettand we spent hours in cafes jamming on the ideas that became the backbone of the Commons Stack.
Those discussions have gone from theoretical considerations to practical concerns. I saw this tweet today:
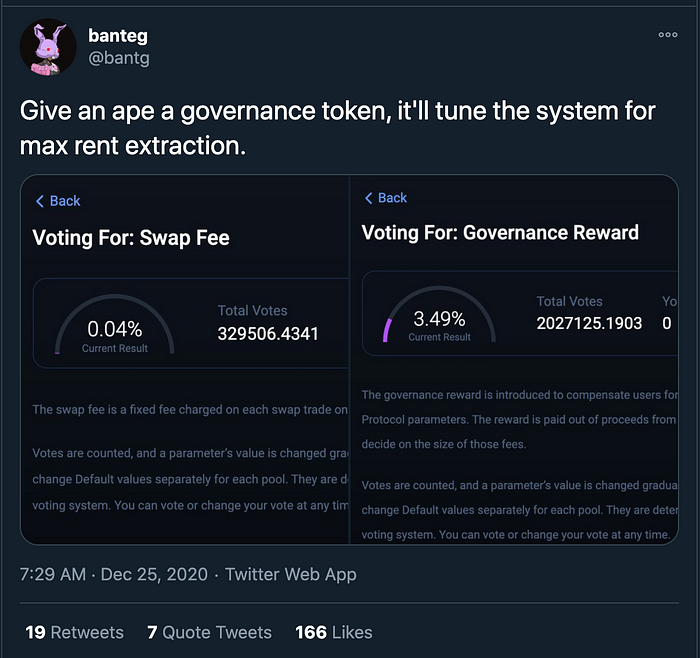
The reason the Commons Stack exists, and the reason the focus has been more on norm formation, understanding boundaries of communities in terms of culture, and teaching principles carried forward from Ostrom’s work is exactly this observation. Governance mechanisms don’t do anything directly, they merely convert the signals expressed by their participants into a collective decision. Acknowledging that value is inter-subjective, it is incredibly important to reflect on what values you express through your participation in governance processes — and the extent to which your community shares those value determines the direction your governance process steers your system.
To keep this article from getting exceptionally long, I am going to fast forward past some really cool things that happened in the world of governance and algorthmic policy-making. Some highlights though — in February 2019, Gitcoin Launch its first matching round and the MolochDAO was deployed. These are among the longest running social ‘signal processing’ systems in the crypto-space. In early 2019, I was also very involved with SourceCred — a project about which I am very bullish. Again, the key concepts are: algorithms and their parameter selections are subjective choices and how framing these choices in a dynamic game provides insight into governance questions.
The on-going development of cadCAD has been motivated by a desire to use dynamic games as a frame for evaluating mechanisms (and parameters) for their signal processing properties. For Conviction Voting in particular, quite a lot of modeling has taken place both before and after the deployment of the algorithm by the 1hive DAO.
Here is an outline of relevant documentation:
- Participate: join 1hive or Commonstack
- Original Specification: https://github.com/BlockScience/conviction/blob/master/social-sensorfusion.pdf…
- Algorithm Documentation & Simulations: https://github.com/1Hive/conviction-voting-cadcad/blob/master/README.md…
- Solidity Implementation:
https://github.com/1Hive/conviction-voting-app…
Discussion on Decentralized Organizations w/ @Sys_innovation & @jeffemmett
Conviction voting is one of many potential algorithms to help bridge the gap between the subjective realm of policy making. You can read more about my research on governance:
- Foundations of Cryptoeconomic Systems with Shermin Voshmgir
- Techno-Reflexivity & Algorithms as Policy with Kelsie Nabben
- Towards Computer Aided-Governance of Gitcoin Grants, with Jeff Emmett and Danilo Lessa Bernardineli
- Introduction to Govbase with Joshua Tan
- Exploring DAOs as a new kind of Institution with Joshua Tan, Primavera de Filippi and Jeff Emmett
I have a range on-going research projects, including computer aided governance in the Filecoin Ecosystem, DAO-to-DAO interaction patterns with
Curve Labs, and broader questions about governance of online spaces with Metagov.org. Specifically on the topic of time-weighted voting, the conviction voting work is currently focused on collecting and analyzing data from the 1hive DAO. Other researchers in space have addressed time weighting, eg, Commitment Voting, Chris Berg, Sinclair Davidson and Jason Potts.
About BlockScience
BlockScience® is a complex systems engineering, R&D, and analytics firm. Our goal is to combine academic-grade research with advanced mathematical and computational engineering to design safe and resilient socio-technical systems. We provide engineering, design, and analytics services to a wide range of clients, including for-profit, non-profit, academic, and government organizations, and contribute to open-source research and software development.